你對世界了解多少很瘋狂。您了解我們生活在3D環境中,物體移動,人們交談,動物飛翔。世界上有大量的數據,其中大部分都很容易獲取 – 困難的部分是開發能夠分析和理解這些豐富數據的算法。生成模型是實現這一目標的最有前途的方法之一。生成模型有許多短期應用,但從長遠來看,它們有可能學習數據集的自然特徵,無論是類別,像素,音頻樣本還是完全不同的東西。
生成算法
您可以將生成算法分組到三個桶中的一個:
- 鑒於標籤,他們預測相關的功能(樸素貝葉斯)
- 給定隱藏的表示,他們預測相關的特徵(變分自動編碼器,生成對抗網絡)
- 鑒於一些功能,他們預測其餘的(修復,插補)
在這篇文章中,我們將探索生成對抗網絡(GAN)的一些基礎知識!GAN具有令人難以置信的潛力,因為他們可以學習模仿任何數據分佈。也就是說,GAN可以學習在任何領域創造類似於我們自己的世界:圖像,音樂,語音。
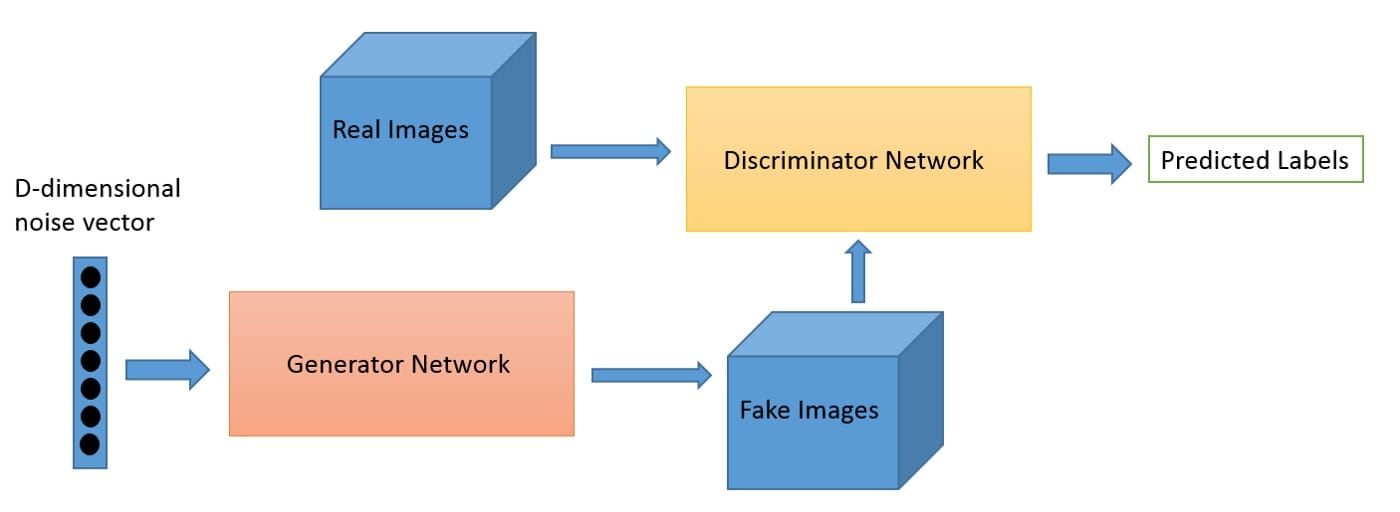
生成對抗網絡(GAN)
「生成」部分
- 叫做發電機。
- 給定某個標籤,嘗試預測功能。
- EX:鑒於電子郵件被標記為垃圾郵件,預測(生成)電子郵件的文本。
- 生成模型學習各個類的分佈。
「對抗性」部分
- 稱為判別者。
- 鑒於這些功能,嘗試預測標籤。
- EX:根據電子郵件的文本,預測(區分)垃圾郵件或非垃圾郵件。
- 判別模型學習了類之間的界限。
GAN如何運作?
一個稱為Generator的神經網絡生成新的數據實例,而另一個神經網絡Discriminator則評估它們的真實性。
您可以將GAN視為偽造者(發電機)和警察(Discriminator)之間的貓捉老鼠遊戲。偽造者正在學習製造假錢,警察正在學習如何檢測假錢。他們都在學習和提高。偽造者不斷學習創造更好的假貨,並且警察在檢測它們時不斷變得更好。最終的結果是,偽造者(發電機)現在接受了培訓,可以創造出超現實的金錢!
讓我們用MNIST手寫數字數據集探索一個具體的例子:
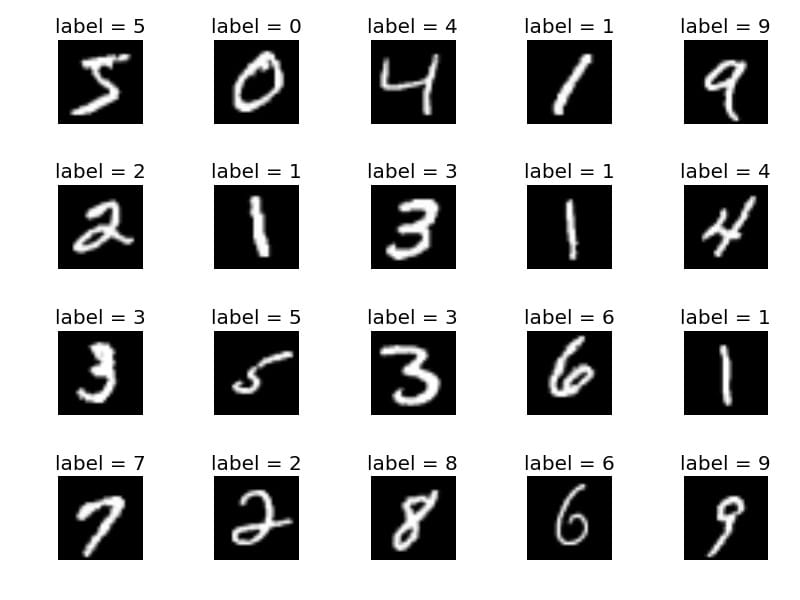
我們將讓Generator創建新的圖像,如MNIST數據集中的圖像,它取自現實世界。當從真實的MNIST數據集中顯示實例時,Discriminator的目標是將它們識別為真實的。
同時,Generator正在創建傳遞給Discriminator的新圖像。它是這樣做的,希望它們也將被認為是真實的,即使它們是假的。Generator的目標是生成可通過的手寫數字,以便在不被捕獲的情況下進行說謊。Discriminator的目標是將來自Generator的圖像分類為假的。
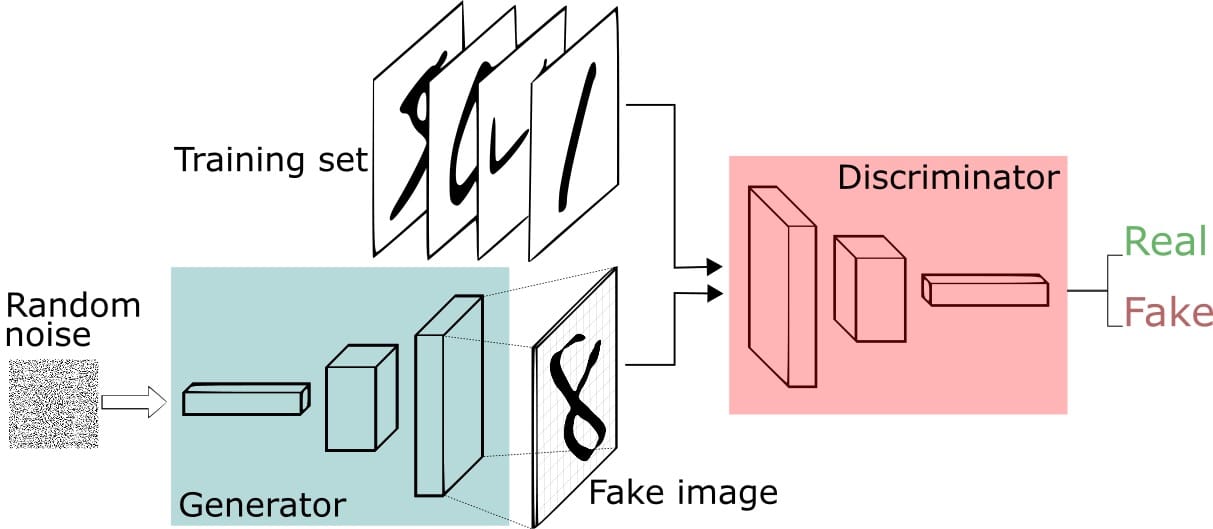
GAN步驟:
- 生成器接收隨機數並返回圖像。
- 將生成的圖像與從實際數據集中獲取的圖像流一起饋送到鑒別器中。
- 鑒別器接收真實和假圖像並返回概率,0到1之間的數字,1表示真實性的預測,0表示假。
兩個反饋循環:
- 鑒別器處於反饋循環中,具有圖像的基本事實(它們是真實的還是假的),我們知道。
- 發生器與Discriminator處於反饋循環中(Discriminator將其標記為真實或偽造,無論事實如何)。
培訓GAN的技巧?
在開始訓練發生器之前預先識別鑒別器將建立更清晰的梯度。
訓練Discriminator時,保持Generator值不變。訓練發生器時,保持Discriminator值不變。這使網絡能夠更好地了解它必須學習的梯度。
GAN被制定為兩個網絡之間的遊戲,重要(並且很難!)保持它們的平衡。如果發電機或鑒別器太好,GAN可能很難學習。
GAN需要很長時間才能訓練。在單個GPU上,GAN可能需要數小時,在單個CPU上,GAN可能需要數天。
GAN代碼示例
足夠的話。以下是由Erik Linder創建的Keras實施的GAN示例:
class GAN():
def __init__(self):
self.img_rows = 28
self.img_cols = 28
self.channels = 1
self.img_shape = (self.img_rows, self.img_cols, self.channels)
optimizer = Adam(0.0002, 0.5)
# Build and compile the discriminator
self.discriminator = self.build_discriminator()
self.discriminator.compile(loss='binary_crossentropy',
optimizer=optimizer,
metrics=['accuracy'])
# Build and compile the generator
self.generator = self.build_generator()
self.generator.compile(loss='binary_crossentropy', optimizer=optimizer)
# The generator takes noise as input and generated imgs
z = Input(shape=(100,))
img = self.generator(z)
# For the combined model we will only train the generator
self.discriminator.trainable = False
# The valid takes generated images as input and determines validity
valid = self.discriminator(img)
# The combined model (stacked generator and discriminator) takes
# noise as input => generates images => determines validity
self.combined = Model(z, valid)
self.combined.compile(loss='binary_crossentropy', optimizer=optimizer)
def build_generator(self):
noise_shape = (100,)
model = Sequential()
model.add(Dense(256, input_shape=noise_shape))
model.add(LeakyReLU(alpha=0.2))
model.add(BatchNormalization(momentum=0.8))
model.add(Dense(512))
model.add(LeakyReLU(alpha=0.2))
model.add(BatchNormalization(momentum=0.8))
model.add(Dense(1024))
model.add(LeakyReLU(alpha=0.2))
model.add(BatchNormalization(momentum=0.8))
model.add(Dense(np.prod(self.img_shape), activation='tanh'))
model.add(Reshape(self.img_shape))
model.summary()
noise = Input(shape=noise_shape)
img = model(noise)
return Model(noise, img)
def build_discriminator(self):
img_shape = (self.img_rows, self.img_cols, self.channels)
model = Sequential()
model.add(Flatten(input_shape=img_shape))
model.add(Dense(512))
model.add(LeakyReLU(alpha=0.2))
model.add(Dense(256))
model.add(LeakyReLU(alpha=0.2))
model.add(Dense(1, activation='sigmoid'))
model.summary()
img = Input(shape=img_shape)
validity = model(img)
return Model(img, validity)
def train(self, epochs, batch_size=128, save_interval=50):
# Load the dataset
(X_train, _), (_, _) = mnist.load_data()
# Rescale -1 to 1
X_train = (X_train.astype(np.float32) - 127.5) / 127.5
X_train = np.expand_dims(X_train, axis=3)
half_batch = int(batch_size / 2)
for epoch in range(epochs):
# ---------------------
# Train Discriminator
# ---------------------
# Select a random half batch of images
idx = np.random.randint(0, X_train.shape[0], half_batch)
imgs = X_train[idx]
noise = np.random.normal(0, 1, (half_batch, 100))
# Generate a half batch of new images
gen_imgs = self.generator.predict(noise)
# Train the discriminator
d_loss_real = self.discriminator.train_on_batch(imgs, np.ones((half_batch, 1)))
d_loss_fake = self.discriminator.train_on_batch(gen_imgs, np.zeros((half_batch, 1)))
d_loss = 0.5 * np.add(d_loss_real, d_loss_fake)
# ---------------------
# Train Generator
# ---------------------
noise = np.random.normal(0, 1, (batch_size, 100))
# The generator wants the discriminator to label the generated samples
# as valid (ones)
valid_y = np.array([1] * batch_size)
# Train the generator
g_loss = self.combined.train_on_batch(noise, valid_y)
# Plot the progress
print ("%d [D loss: %f, acc.: %.2f%%] [G loss: %f]" % (epoch, d_loss[0], 100*d_loss[1], g_loss))
# If at save interval => save generated image samples
if epoch % save_interval == 0:
self.save_imgs(epoch)
def save_imgs(self, epoch):
r, c = 5, 5
noise = np.random.normal(0, 1, (r * c, 100))
gen_imgs = self.generator.predict(noise)
# Rescale images 0 - 1
gen_imgs = 0.5 * gen_imgs + 0.5
fig, axs = plt.subplots(r, c)
cnt = 0
for i in range(r):
for j in range(c):
axs[i,j].imshow(gen_imgs[cnt, :,:,0], cmap='gray')
axs[i,j].axis('off')
cnt += 1
fig.savefig("gan/images/mnist_%d.png" % epoch)
plt.close()
if __name__ == '__main__':
gan = GAN()
gan.train(epochs=30000, batch_size=32, save_interval=200)
如何改善GAN?
GAN剛剛在2014年發明 – 它們非常新!GAN是一個很有前途的生成模型家族,因為與其他方法不同,它們可以生成非常乾淨和清晰的圖像,並學習包含有關基礎數據的有價值信息的權重。但是,如上所述,可能難以使Discriminator和Generator網絡保持平衡。有很多正在進行的工作使GAN培訓更加穩定。
除了生成漂亮的圖片之外,還開發了一種利用GAN進行半監督學習的方法,該方法涉及鑒別器產生指示輸入標籤的附加輸出。這種方法可以使用極少數標記示例在數據集上實現最前沿結果。例如,在MNIST上,通過完全連接的神經網絡,每個類只有10個標記示例,實現了99.1%的準確度 – 這一結果非常接近使用所有60,000個標記示例的完全監督方法的最佳已知結果。這是非常有希望的,因為在實踐中獲得標記的示例可能非常昂貴。
結論
GAN仍然是如此新鮮 – 我很高興看到他們去了哪裡!不是嗎?
本文轉自towardsdatascience,原文地址
7 Comments
這機翻…
有些內容的確是機翻的,不過機翻的內容也是經過篩選的,不是隨便找些內容機翻一下湊數
真的很不錯呢!
原文好像被刪了,請問有什麼辦法可以看到嗎
被刪除了我也沒辦法了
原文好像被刪了,請問有什麼辦法可以看到嗎
原文不是我寫的,所以我也沒辦法